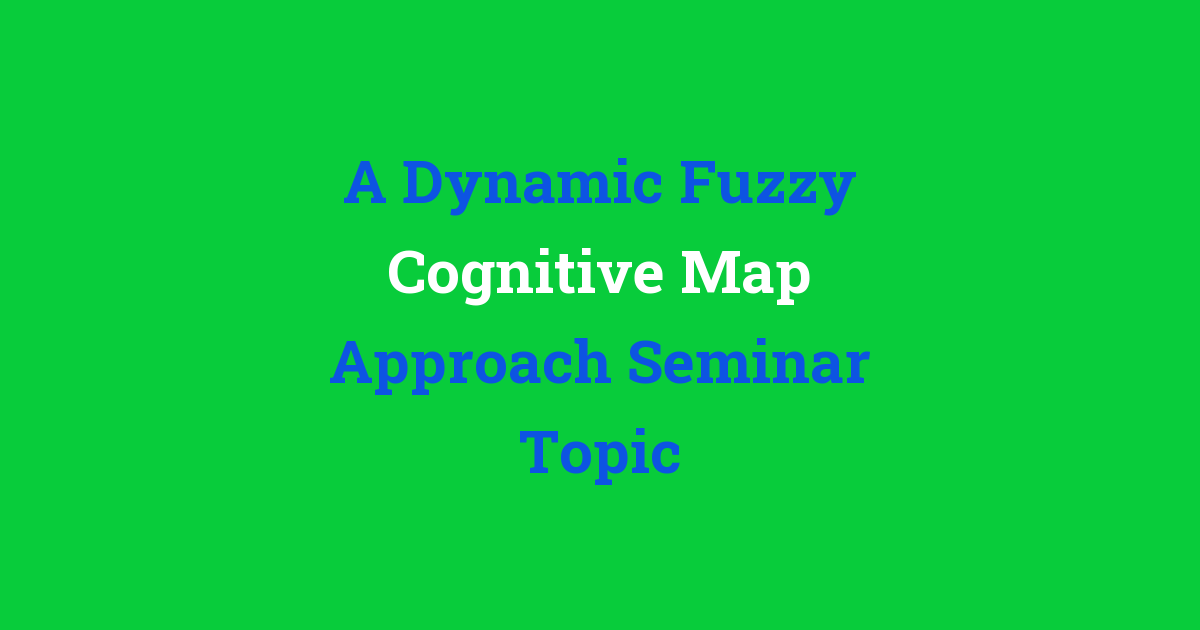
“Exploring the Impact of Artificial Intelligence on Global Business Trends”
Introduction
Engineering is a constantly evolving field, with new technologies and methodologies being developed every day. One such advanced concept that has gained recent attention is the Dynamic Fuzzy Cognitive Map approach. This innovative approach combines fuzzy logic and cognitive mapping to create a dynamic system that can model complex relationships between variables in a system. In this seminar topic, we will explore the basics of Dynamic Fuzzy Cognitive Maps and how they can be applied in various engineering fields.
Problem Statement
In traditional engineering systems, it can be challenging to accurately model the complex, dynamic relationships between variables. This can lead to inaccurate predictions and suboptimal decision-making. The existing systems often rely on static models that do not account for the changing nature of the system. This limitation has been a significant challenge in various engineering fields, from mechanical engineering to computer science.
Existing System
In the existing system, engineers typically use static models to analyze and predict the behavior of a system. These models are based on crisp logic, which can be limiting when dealing with uncertainty and imprecision in the data. Additionally, these models do not account for the dynamic nature of the system, leading to inaccurate results. As a result, decision-making in engineering fields can be suboptimal, and the systems may not perform as expected.
Disadvantages
The traditional static models used in engineering have several disadvantages. Firstly, they do not account for uncertainty and imprecision in the data, leading to inaccurate predictions. Secondly, these models do not capture the dynamic relationships between variables, which are crucial for understanding complex systems. Finally, the reliance on crisp logic can be limiting when dealing with the real-world complexity of engineering problems.
Proposed System
The Dynamic Fuzzy Cognitive Map approach offers a new way to model complex systems in engineering. This approach combines fuzzy logic and cognitive mapping to create a dynamic system that can adapt to changes in the environment. By using fuzzy logic, the system can handle uncertainty and imprecision in the data, leading to more accurate predictions. Additionally, the dynamic nature of the system allows it to capture the changing relationships between variables, providing a more realistic model of the system.
Advantages
There are several advantages to using the Dynamic Fuzzy Cognitive Map approach in engineering. Firstly, this approach can handle uncertainty and imprecision in the data, leading to more accurate predictions. Secondly, the dynamic nature of the system allows it to adapt to changes in the environment, making it more robust. Finally, the ability to model complex relationships between variables can lead to better decision-making and improved system performance.
Features
The Dynamic Fuzzy Cognitive Map approach offers several key features that make it a valuable tool in engineering. Firstly, the use of fuzzy logic allows the system to handle uncertainty and imprecision in the data. This is crucial for real-world engineering problems, where data may be incomplete or noisy. Secondly, the dynamic nature of the system enables it to adapt to changes in the environment, ensuring that the model remains accurate over time. Finally, the ability to model complex relationships between variables allows engineers to gain a deeper understanding of the system and make more informed decisions.
Conclusion
In conclusion, the Dynamic Fuzzy Cognitive Map approach is a promising new technique that can revolutionize the way engineers model complex systems. By combining fuzzy logic and cognitive mapping, this approach offers a more robust and accurate way to understand the relationships between variables in a system. With its ability to handle uncertainty, adapt to changes, and model complex relationships, the Dynamic Fuzzy Cognitive Map approach has the potential to improve decision-making and system performance in various engineering fields. This seminar topic provides a glimpse into the exciting possibilities that this approach offers and highlights the need for further research and development in this area.